Q2’24: AI – Singularity or Dot-Com-Sequel?
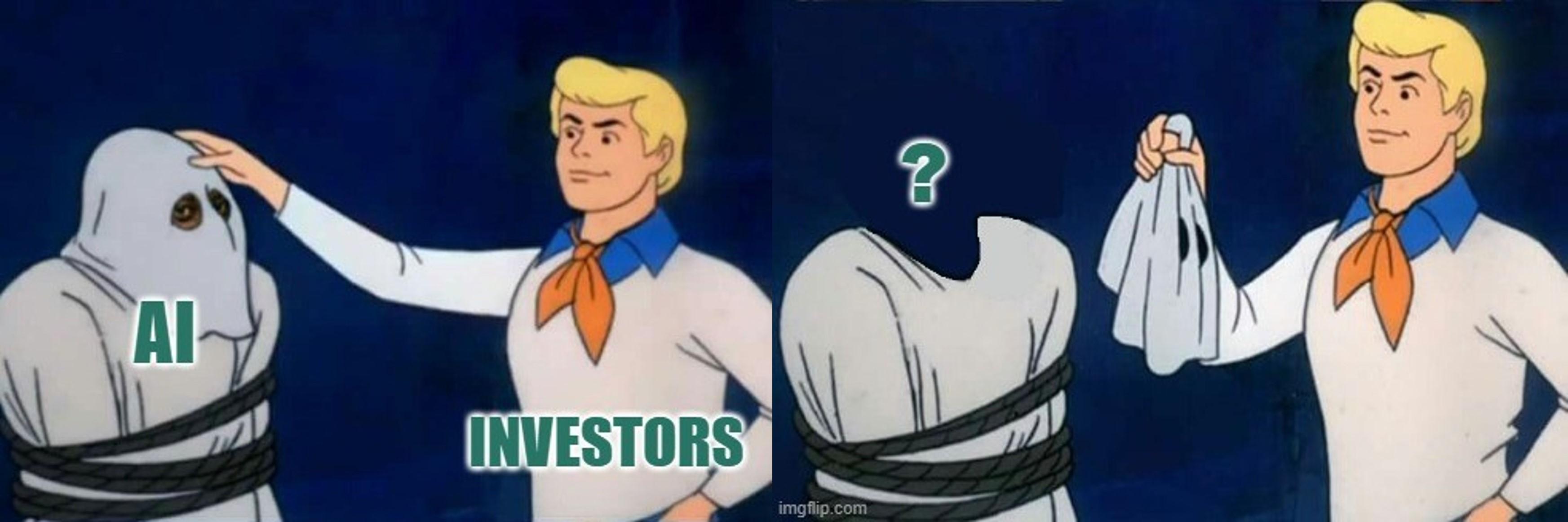
By: Jack Schibli
As a reminder, this blog is an outlet for our thoughts, primarily on the macroeconomic environment, which contextualizes our investments. Please subscribe via the form at the bottom of the page to receive future post notifications.
If you are interested in learning more about our investment strategies, please kindly fill out our contact form here.
TLDR: – "too long; didn’t read." We recognize our posts can be lengthy and challenging to digest, so here’s our executive summary:
- Lead technologists are driving artificial intelligence towards a futuristic, possibly dystopian, society filled with massive innovation potential but equally massive risks.
- The recent AI infrastructure surge is akin to a modern-era Space Race to stockpile compute capability and accelerate AI model development.
- The near-term economic use cases of AI models are less clear, and their revenue generating potential a major question mark for those requiring a return on investment.
- AI models in their current state have fundamental flaws that limit real-world usability.
- Nvidia, and more specifically, its stock price, faces an uphill battle of inflated expectations with a number of risks.
- AI’s disruption generates more questions than answers, but one thing is certain – the genie is out of the bottle, and humankind is marching toward greater integration with machines.
To have an opinion on the stock market today is to have an opinion on AI. We have spent plenty of ink writing about the increasing concentration in stock market indices, which now stands at all-time highs. As of the second quarter end, the top 3 stocks in the S&P 500 (“the market”) accounted for 20% of the index, the top 10 accounts for 37% and the top 50 accounts for 60% of the index – so much for diversification. The AI narrative has driven mega-cap tech stocks to materially outperform virtually anything else. When combined with the passive investing phenomenon, this outperformance accelerates the dispersion of returns within the index – effectively, the big get bigger at the expense of everything else. (Today’s topic is AI, but if you’d like to read more about the passive phenomenon, check out this post).
Let’s dive into the past, present, and future of artificial intelligence.
The Modern Space Race 🌓
The term artificial intelligence was first coined by John McCarthy in 1955 to articulate the concept that machines could simulate human-level intelligence. He organized a conference at Dartmouth College that would kick off the ongoing, decades-long journey to replicate the biological brain with 1s and 0s. It wasn’t until the early 1990s, as computational power grew dramatically, that early applications of “AI” gained traction. Machine learning emerged as the most popular subcategory of AI research, utilizing algorithms to comb large data sets, identify patterns, and make predictions. Companies have been employing machine learning algorithms for decades; some everyday examples include the Google search algorithm or Meta’s advertising algorithm.
Most of these initial AI technologies were undetectable to the everyday person, but that all changed after OpenAI released ChatGPT in late 2022. ChatGPT utilizes generative AI (GenAI) – the GPT stands for Generative Pre-trained Transformer – which employs transformer-based neural networks to, as the name implies, “pre-train” on large data sets and draw on them to produce the most relevant output using a predictive model technique called natural language generation. Importantly, ChatGPT was the first consumer-facing GenAI application, which catalyzed and defined the current AI movement that has incorrectly synonymized AI with GenAI. ChatGPT added 100 million users in just two months, far outpacing the previous record for such a feat held by TikTok (nine months).
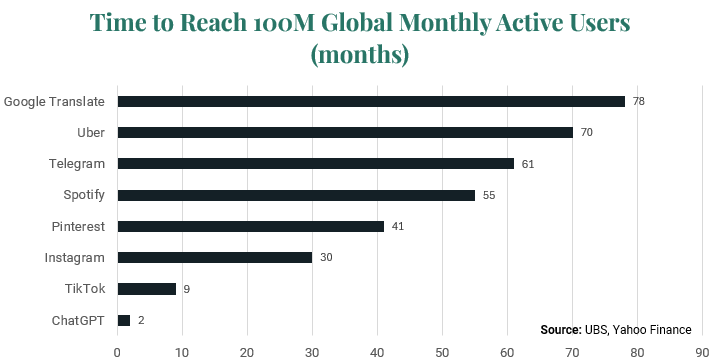
Users will recall that feeling of awe when first interacting with ChatGPT or the many other Large Language Models (LLMs) that quickly made their way to market. Watching these models complete tasks like writing Shakespearean sonnets on a topic of your choosing seems nothing short of magical. LLMs’ overnight sensation sowed the seeds of a modern-era Space Race, an all-out scramble for compute capability in search of John McCarthy’s 1955 vision for true artificial intelligence – a concept now referred to as AGI (Artificial General Intelligence) to denote when AI reaches human-level capabilities. In response, cloud computing providers, known as hyperscalers – the likes of Amazon, Microsoft, Google, etc., dove headfirst into AI infrastructure investment. The law of large numbers makes the growth of trillion-dollar businesses hard to come by, so hyperscalers are hopeful AI will generate outsized growth to offset their maturing core businesses.
To understand the motivations of these large tech companies, we first must understand what’s at stake. In what reads as a manifesto, AI “insider” Leopold Aschenbrenner authors the somewhat dystopian view of Silicon Valley evangelists and their search for “superintelligence.” Also referred to as ASI (artificial superintelligence), it would be as if the top human minds were mere preschoolers amidst a fleet of advanced PhD-level machine intelligence. Here are some of the noteworthy ASI predictions – the good, bad, and ugly:
- Fleets of hundreds of millions of GPUs could run a civilization of billions of AI agents, all thinking orders of magnitude faster than humans.
- Compress a century of research and development progress into a few years, advancing robotics, science, technology, and medicine breakthroughs – a Cambrian explosion of innovation.
- Factories would go from human-run to AI-directed human labor to fully automated swarms of robots.
- Generate new means of communication and languages that humans cannot interpret, limiting our ability to police them.
- Develop advanced military systems, including drone swarms, robot armies, new types of weaponry, novel WMDs, and bioweapons.
- Possibly overthrow the U.S. government through hacking, election interference, and media control and distortion.
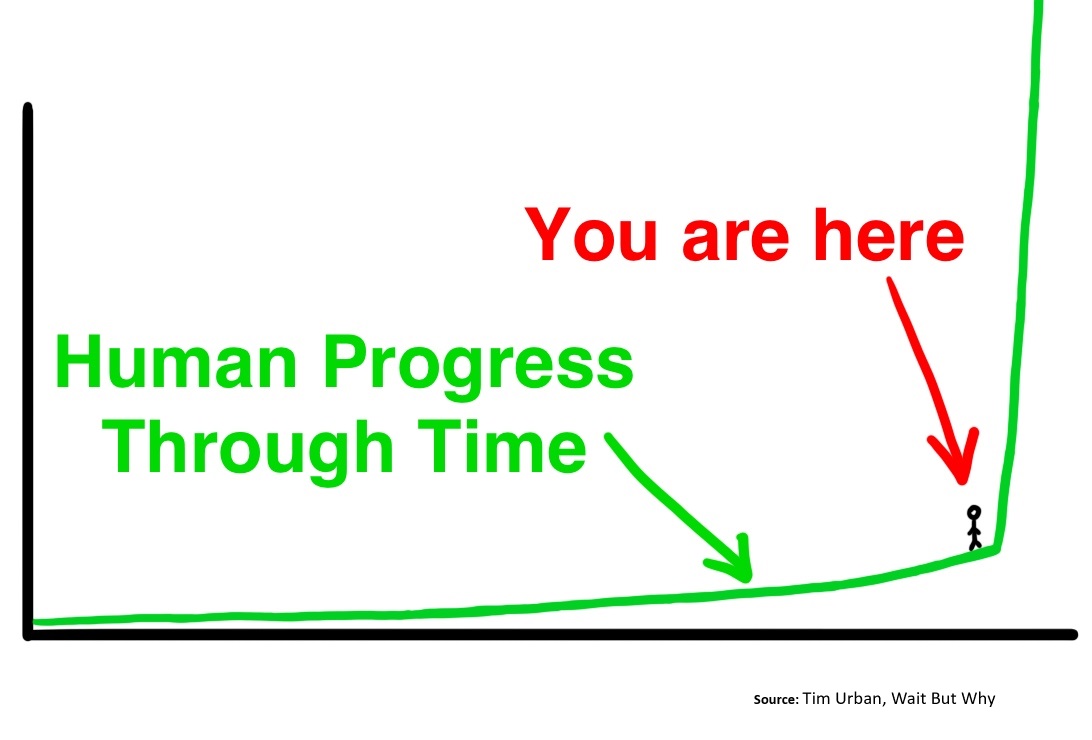
Assuredly, this would be the most volatile period in human history. ASI sounds like a sci-fi novel, but the scariest part is leading AI researchers' view its emergence as simply a matter of time with leading estimates as soon as 2030. Their game plan is first to reach AGI, the equivalent of a “plug-and-play” human knowledge worker, and then use it to displace their own jobs as AI researchers, presumably enabling a step change in model development ability. The going theory is that “scaling laws” – a power law relationship between the performance of AI models and the amount of compute, number of parameters, and size of training data set used – will hold as they have in recent years.
This assumes effective compute gains of six orders of magnitude (10^6) by 2029, or a one million times improvement. There is a laundry list of physical constraints to confront[1]:- Data – These LLMs are trained on ever-increasing amounts of data, and we’ve already given the most current models access to the entire internet – data can’t continue to scale exponentially.
- Compute—Sam Altman, CEO of OpenAI, is proposing a $1 trillion cluster of GPUs for training LLMs; this is the scale required to continue the aforementioned scaling law—but who can afford to spend nearly 4% of US GDP?
- Power—Aschenbrenner estimates that total AI electricity demand will equal total US electricity generation in 2030. Where will we find an entire nation’s worth of power in just six years?
- Chips – Taiwan Semiconductor Co. (TSMC) produces most of the world’s AI chips, currently amounting to ~10% of TSMC production. To meet the 2030 ASI projections, TSMC would need dozens more Gigafabs, and they are struggling to build just one over several years in Arizona.
AI evangelists believe these are not insurmountable.
Lastly, it’s important to mention the risks associated with seeking ASI, which Aschenbrenner is acutely aware of as a former member of the “superalignment” team at OpenAI. His job was to devise ways to keep AI “aligned” with humans as it scales toward ASI, which current techniques would fail to accomplish. We must worry not only about our ability to control a superintelligent system but also about human access to such powerful technology. In the wrong hands, ASI could be catastrophic, yet there is currently little to no security at leading AI labs. A finished AI model is simply a file on a computer and can be utilized with a fraction of the computing power that it takes to train the model. Venture capitalist Marc Andreessen recently said, “[We see] the security equivalent of Swiss cheese…My own assumption is that all such American AI labs are fully penetrated and that China is getting nightly downloads of all American AI research and code right now.” If superintelligence will be the world’s most powerful weapon ever created, why are we not treating it like Los Alamos 2.0?
Where the Rubber Meets the Road 🛣️
With superintelligence driving the AI space race as context, let’s get into the math behind current AI investment and its required returns in the near term. The hyperscalers have undertaken the largest investment cycle in their history and will soon need to generate returns on these investments by re-selling the AI compute to their cloud customers, and that's when the questions start to stack up. We can use some napkin math methodology used by David Cahn of Sequoia Venture Capital to build a view of AI capital expenditures based on Street estimates for Nvidia data center revenues – the bellwether of AI [more on this later]. The assumptions are that Nvidia GPUs are roughly half the overall cost of the data center buildout, an oft-cited rule of thumb and that hyperscalers will seek a 50% margin.
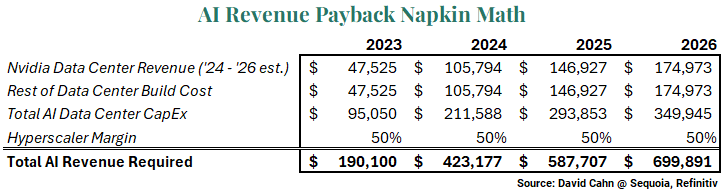
This estimate implies nearly $700 billion in lifetime revenues required for each year of CapEx at 2026 levels. Yet, realized AI revenues are developing far slower than expected. OpenAI, the center of this fervor, is running at $3.4 billion in annual revenues, up from $1.6 billion in late 2023. Deduced based on first quarter’s earnings, Microsoft’s Azure cloud AI revenue is running at about $1.25 billion, plus their CoPilot offering is contributing about $650 million for a cumulative run rate of under $2 billion (3% of total revenue)[2]. Very generously assuming Microsoft can scale this 10x to $20 billion in 2026 and that OpenAI and their hyperscaler peers (Amazon, Google, Meta) can do that same, we get ~$100 billion. Elsewhere in the ecosystem, let’s assume second-tier players Oracle, X (formerly Twitter), Anthropic, Tesla, Alibaba, and a few more might generate half of their larger peers ($10 billion each). Add on some incremental revenue from new and scaling AI startups, and we are in the $200 billion dollar range, far short of the required revenue hurdle and well below the cost of data center buildouts. These are admittedly extremely rough estimates, but the focal point is the buildout cost compared to anticipated near-term revenues are in different zip codes, hundreds of billions apart – thanks again to David Cahn at Sequioa for this framework.
Amidst enterprise board rooms, we believe there is general consensus that AI will be revolutionary, but the current actionable use cases are less clear. A recent survey by Lucidworks found that only 63% of global companies plan to increase AI spending in the next 12 months, compared to 93% last year. The financial services sector appears to be finding the best use cases, with 70% of firms planning increased spending. 42% of companies have yet to see a significant benefit from existing GenAI initiatives, with hesitancy around costs rising most dramatically, as 43% cited concerns versus 3% last year. Just one in eight companies saw revenue growth contribution, and just one in six saw operating expense reduction. The hyperscalers are hoping their customers figure it out quickly. Soon, the depreciation of this investment cycle will hit their income statement, and they would like to have some material offsetting revenue to show for it. An under-discussed phenomenon is the transition of these large-cap technology companies from capital-light businesses to capital-intensive.
One of the impediments to adoption might be fundamental architectural flaws of LLMs. Very simplistically, LLMs are trained on massive sets of data, use statistical models to analyze language patterns, and apply those patterns to probabilistically predict responses one word at a time. To date, most of these LLMs’ performance has been measured by how well they can complete benchmark tests, typically standardized tests like the LSAT, GMAT, or SAT, to go up against human scores. The issue is that inclusive in more and more training data are thousands of examples of these tests. Thus, the models can recognize the problem’s structure, compare it to the training data, and correctly answer a multiple-choice question. Is this intelligence? Francois Chollet, famed AI researcher at Google, thinks otherwise. He believes LLMs are simply memory, not a sign of intelligence. It’s a philosophical question – what is intelligence? His answer is the ability to react and respond to novel experiences – a.k.a. those not found in training data. LLMs are trained on human-created data, which inherently has structure. Yet, so far, LLMs are incapable of learning on unstructured universe data – the type humans encounter and navigate daily.
Chollet has designed a benchmark called ARC, specifically to present novel problems to LLMs in an effort to segment intelligence from knowledge. Humans have no problem passing this test with an average score of 85%; even five-year-olds score over 50%, but the very best model score on ARC is just 35%, and ChatGPT-3 scored a zero[3]. If you’re interested in checking out the ARC test, head here to try a few sample problems – there’s a $1 million prize on the line for anyone who can get their LLM to beat it.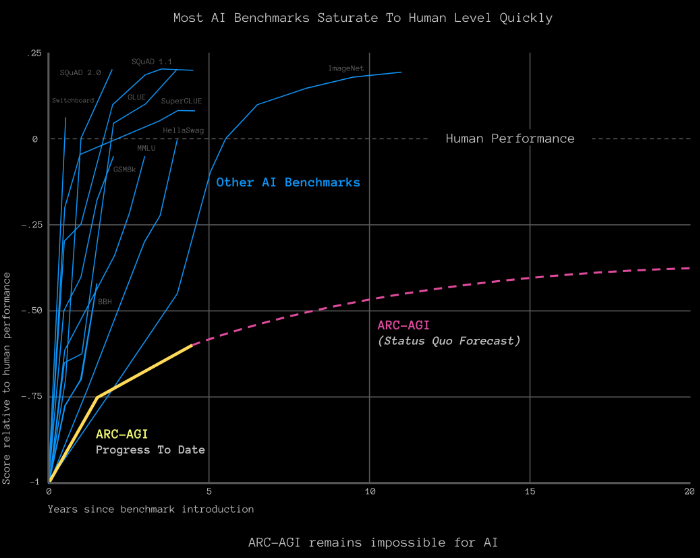
LLMs can theoretically automate anything with a static distribution since they can be trained on it or problems with similar reasoning patterns, but they cannot yet adapt to change. For example, if you took one of the self-driving cars currently operating in San Francisco and dropped it in New York City, the car couldn’t drive. Years of driving data have mapped the San Francisco streets, but the model cannot generalize its learnings the way a human who learns to drive in one city can then drive around the world. Consequently, current LLM use cases and value creation remain limited. In large part, because even when applied to static distribution tasks, the models responses cannot be relied upon due to what’s called “hallucination” – the generation of false or misleading information. We don’t believe these challenges are insurmountable, but these models need time and advancement to display a level of maturity that enterprises are willing to put into production at scale.For Illustrative Purposes Only
Despite being foundationally built on math, LLMs are not math whizzes. However, they are exceptionally creative – they can impressively generate copy, poems, art, videos, etc. We believe creative use cases continue to be the most prominent. Akin to the launch of the PC when the marginal cost of compute fell towards zero, and the emergence of the internet when the marginal cost of distribution fell towards zero, LLMs are likely to drive the marginal cost of creative content towards zero.
Heart of the Bubble 🫧
Now a household name, Nvidia has been the main beneficiary of AI infrastructure spending, selling the GPUs (Graphics Processing Units) that enable LLM training. In June, Nvidia surpassed Microsoft to become the world’s largest company, valued at $3.3 trillion – though the title lasted just a single day. It’s worth diving into the fragility surrounding this valuation and potential risks.
Nvidia’s quasi-monopoly lies not necessarily with the chips themselves but with its proprietary software program (CUDA) that coordinates the use of thousands of GPUs in parallel to train a singular model. Competitors, like AMD, now have chips that outperform Nvidia on a standalone basis, but when accounting for the difficulty of transcribing CUDA instructions to AMD’s open-source version (ROCm), the performance and cost is inferior.
Following ChatGPT’s lightbulb moment, demand soon overwhelmed supply, and shortages ensued, with lead times reaching nearly 12 months. Importantly, this overwhelming demand for Nvidia’s short-supply product meant Nvidia could raise prices, dramatically increasing its gross margins, which have jumped to 79% from historical levels of 55-60%. Commensurately, Nvidia’s dramatic revenue growth of 126% far outpaced unit volume growth of just 42%. We highlight this margin expansion because, at some point, it will reverse, disproportionately affecting Nvidia’s earnings – the same way they have been disproportionately affected to the upside.- Competition - "your margin is my opportunity" - long-time rival AMD won't sit pat, and neither will the hyperscalers (all developing their own ASICs to reduce Nvidia dependence), nor any of the very well-funded AI-specific chip start-ups (Groq, Cerebras, and Etched to name a few).
- Critical supplier reliance - Taiwan Semiconductor (TSMC), who manufactures all of Nvidia's chips, is also looking at their margin, saying, "Hey, I’ll be taking some of that" (early evidence).
- Shortages are typically followed by surpluses and surpluses mean prices come down. Many startups have acquired large hoards of GPUs, such as Coreweave and Lambda Labs, with far less proven business models and robust customer bases when compared to hyperscalers. Those GPUs could flood the secondary market if they cannot generate sustainable revenues.
Any way you slice it, margins look vulnerable, and when you assume, perhaps generously, a gross margin of 60% run through Nvidia's P&L on the Streets 2027 revenue estimate of $230 billion, earnings per share comes out to $2.96, just 9% higher than this years estimate. Did we mention the forward P/E ratio is 45x? We did not even account for revenue falling short of expectations, which it naturally would if ASPs came down or any of the competitive threats materialized.
To add more fuel to the downside case, AI model and chip innovation may render the GPU an inefficient chip for AI use cases. The precedent for this occurred in cryptocurrency mining, as miners originally used GPUs, but they soon designed ASICs (Application-specific integrated circuits) to mine more efficiently. Using them became more expensive than having them lay idle, so massive swaths of GPU flooded the secondary market, crashing prices and Nvidia sales in 2018. A similar decrease in demand could result from the shift from training to inference. Inference is the process of running an already trained model, and in this arena, Nvidia’s moat is far less defensible, as competing chips and hyperscaler ASICs are already matching it on a performance per dollar basis. Perhaps, Nvidia will continue its impeccable execution, perennially out-innovating the competition. A new hurdle for continued innovation might be the ability to motivate its employees, as according to a recent poll, more than 70% are freshly minted millionaires.
Bubbles tend to last longer than anyone expects, so perhaps this one keeps rolling.
What's Next? 🎱
Based on the current state of LLMs, we believe the technology isn’t ready for mass deployment at the scale that recent and planned AI infrastructure investment requires. This incredibly fast supply cycle is likely to outpace the demand cycle – such a timing mismatch generates an oversupply of compute likely to drive the cost of compute much lower to the detriment of compute providers but to the benefit of the AI researchers – ironically, possibly accelerating their progress. Perhaps similar to the fiber glut in the wake of the dot-com bust in 2001 when 97% of fiber-optic cable lay idle. We do not believe this will end as viciously as the dot-com bust did in aggregate because most (not all) of the companies supporting the AI infrastructure buildout can afford to take the balance sheet risk, whereas companies participating in the internet buildout could not.
We think, like the internet, most value eventually will accrue to those building applications on top the AI compute layer. Public markets, for the most part, haven’t been able to forecast what that looks like and have plowed into the AI infrastructure layer instead, as the only possible way to express the AI theme. Declining compute costs will aid this acceleration of new businesses, products, and ultimately, economic productivity.
Emerging technologies often create more questions than answers; here are some of our key considerations and concerns going forward:- What if model advancement underwhelms expectations and, therefore, significantly detracts from the real-world applicability of the technology?
- In focus right now is the performance of ChatGPT 5 – will it be 10x better than ChatGPT 4 as scaling laws predict?
- How do we measure 10x better performance?
- How do AI labs build moats around their models?
- Since the AI frenzy began, frontier AI labs have gone into development secrecy. Before ChatGPT’s launch, all LLM progress was published openly, accelerating industry progress and is a key reason why many models are currently so similar. Today, frontier labs are spending vast sums on training clusters – what if specific labs make breakthroughs while others don’t? Will they be left behind?
- Relatedly, there is some discussion about using techniques, like model distillation, to effectively improve existing models by training on another. As an example, when ChatGPT 5 is launched, open-source models could train themselves on it, self-improving to match its performance, all at a fraction of the training cost OpenAI will have spent on it – implying very little moat around models.
- Francois Challot believes the move towards secrecy will rapidly slow industry progress and estimates a 5 to 10 year delay in achieving AGI versus an open ecosystem.
- Will hardware progress render the current generation of AI chips archaic, and how soon?
- At the onset of emerging technology, product cycles are particularly short in the sense that we think next generation chips will blow current chips out of the water. We can see this with Nvidia’s Blackwell generation chips set to deliver exponentially more compute per dollar – should this continue, it virtually guarantees the obsolescence of current chips.
- This comes on the heels of Microsoft, Amazon, and Google all increasing the useful life estimate of their servers from 3-4 years to 5-6 years prior to their AI investments. We suspect they will need to return to a 3 to 4 year depreciation schedule, further accelerating depreciation’s impact on their income statements.
- An obvious benefit to chip innovation is that some of the absurd power requirement estimates are likely very incorrect, as they are extrapolating current chip specifications.
- With all the concerns about privacy and data security, will most inference move to the edge?
- Edge inference means interacting with AI models on your device (phone, PC, etc.) rather than making an API call to a cloud server.
- This is Apple’s strategy with the next-generation iPhones. Perhaps edge inference will cover a higher percentage of inference workloads than most expect, leading to wildly oversupplied data centers.
- How deflationary is AI over the long-term?
- In the short term, we expect AI might provide an inflationary impulse, as these massive data center buildouts siphon large quantities of electricity driving up power and energy prices.
- However, over the long-term, displacing labor with technology is always deflationary.
- How will China, our main competitor in this modern-era space race, play their hand?
- We have already hamstrung China with sanctions on semiconductor sales into the country, limiting their access to cutting edge chips from Nvidia and their ability to produce them internally as well.
- However, we should not underestimate their ability to catch up very quickly. As we quoted earlier from Marc Andreesen, China is very good at stealing our technology.
- In the lens of the race for ASI, China has every incentive to invade Taiwan, the manufacturing home to all AI chips via TSMC’s foundry. In a swift and calculated move, China could cut off our access to chips and derail our AI progress for many years.
- What does society look like in a world of AGI or ASI?
- AI fundamentally empowers capital over labor, only serving to widen wealth inequality.
- Amidst a devaluation of human labor, does AI create a dystopian welfare state with mass unemployment? This has been a concern in the early stages of every major technology revolution dating back to the power loom, but has yet to materialize – will this time be different?
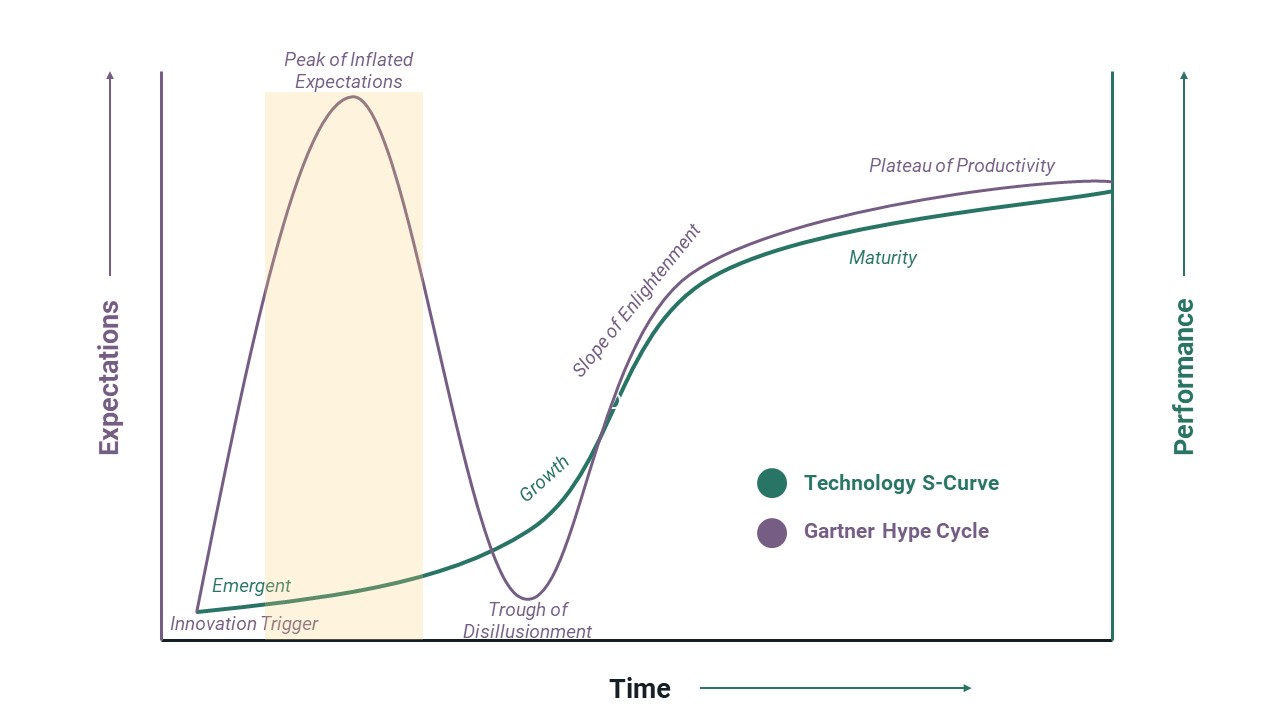
Disclaimers
The information provided is for educational and informational purposes only and does not constitute investment advice and it should not be relied on as such. It should not be considered a solicitation to buy or an offer to sell a security. It does not take into account any investor's particular investment objectives, strategies, tax status, or investment horizon. You should consult your attorney or tax advisor.
The views expressed in this commentary are subject to change based on market and other conditions. These documents may contain certain statements that may be deemed forward‐looking statements. Please note that any such statements are not guarantees of any future performance and actual results or developments may differ materially from those projected. Any projections, market outlooks, or estimates are based upon certain assumptions and should not be construed as indicative of actual events that will occur.
All information has been obtained from sources believed to be reliable, but its accuracy is not guaranteed. There is no representation or warranty as to the current accuracy, reliability, or completeness of, nor liability for, decisions based on such information, and it should not be relied on as such.
Investments involving Bitcoin present unique risks. Consider these risks when evaluating investments involving Bitcoin:
- Not insured. While securities accounts at U.S. brokerage firms are often insured by the Securities Investor Protection Corporation (SIPC) and bank accounts at U.S. banks are often insured by the Federal Deposit Insurance Corporation (FDIC), bitcoins held in a digital wallet or Bitcoin exchange currently do not have similar protections.
- History of volatility. The exchange rate of Bitcoin historically has been very volatile and the exchange rate of Bitcoin could drastically decline. For example, the exchange rate of Bitcoin has dropped more than 50% in a single day. Bitcoin-related investments may be affected by such volatility.
- Government regulation. Bitcoins are not legal tender. Federal, state or foreign governments may restrict the use and exchange of Bitcoin.
- Security concerns. Bitcoin exchanges may stop operating or permanently shut down due to fraud, technical glitches, hackers or malware. Bitcoins also may be stolen by hackers.
- New and developing. As a recent invention, Bitcoin does not have an established track record of credibility and trust. Bitcoin and other virtual currencies are evolving.
No investment strategy or risk management technique can guarantee returns or eliminate risk in any market environment. All investments include a risk of loss that clients should be prepared to bear. The principal risks of Lane Generational strategies are disclosed in the publicly available Form ADV Part 2A.
This report is the intellectual property of Lane Generational, LLC, and may not be reproduced, distributed, or published by any person for any purpose without Lane Generational, LLC’s prior written consent.
For additional information and disclosures, please see our disclosure page.
Sources:
All financial data is sourced from Refinitiv Data or Federal Reserve Economic Data (FRED) unless otherwise noted.
[1] Aschenbrenner, Leopold. Situational Awareness: The Decade Ahead. June 2024
[2] Microsoft Q1 2024 Earnings Report.
[3] Francois Chollet, Mike Knoop – LLMs won’t lead to AGI - $1,000,000 Prize to find true solution. Dwarkesh Podcast. June 11, 2024.